How to add value with AI
Although most major businesses today have started using AI, many are still struggling to get the expected value from the AI-solutions. The main problems are usually:
1. Not a clear picture of how AI should contribute to the business and create real value
2. Not able to integrate the AI-solutions into the established business processes
This blog is about the first problem and where to look for real value.
Baseline
Roughly speaking, one can distinguish between two types of AI:- Rule-based AI - A more or less complicated deterministic regulatory framework where f(input x) = output y regardless of time and context.
- Machine learning – output may change over time and in different contexts because the machine "learns"
"Rule-based AI"
If rule engines can also be AI, how do you distinguish between AI and something as simple as a calculator? The main difference is interpretation. If there is no interpretation at all involved, it is not AI(!) More mathematical: If P(f(x)=y) = 1 (i.e. 100%) where P is probability, then it is not a “AI-result”.
In some AI-based rule engines, it is assumed on a daily basis that P=1, for instance fingerprint or facial recognition on the mobile, but in this type of solution P will never be completely 1 and it is important to be aware that all AI solutions by definition will have a margin of error.
A computer scientist will typically work to get P as close to 1 as possible.
The definition is also applicable to physical robots, this must be able to interpret the situation at a certain level and act accordingly, otherwise it is just a traditional machine.
"Machine learning"
Many people probably equate AI with ML, this is undoubtedly the most well-known and "exciting" part of AI. The fact that the machine learns over time and can thus change output even if the input is similar gives the AI models a kind of "intelligent" dimension that traditional rule motors lack and are therefore considered to have a more of a "human" intelligence.
The ML-model will often itself work to improve P, this is the actual “learning”.
In purely business terms, the distinction between ML and advanced analytics or rule engines is often difficult to see (and in fact often uninteresting), the important thing is that it results in value through increased insight.
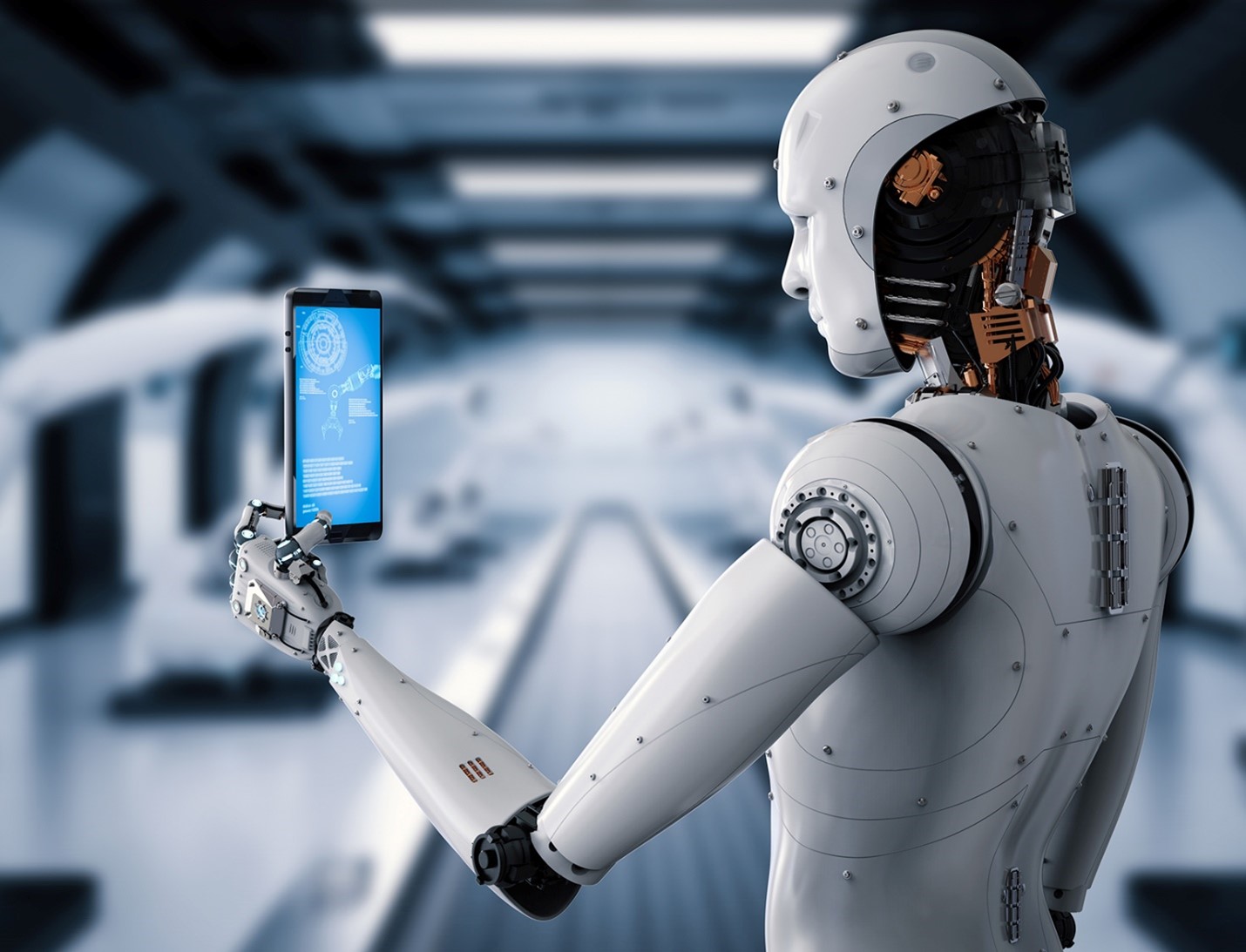
Usage
As consultants, we often experience customers asking us to help them get started with AI without a clear idea of what they intend to use it for. But they "know" that AI is meant to give a competitive advantage and does not want to lose in the competition because they were too late to adopt it.
But is it a given that AI will actually provide value to all types of businesses?
First, let's look at what areas AI can actually contribute.
We can roughly divide it into
- Automation
- Data recognition and categorization
- Unstructured data such as images, sound and text
- Large and cluttered amounts of data ("Big Data")
- Case management (e.g. through Robotic Process Automation)
- Physical robots
- Data recognition and categorization
- Insight
- Internal prediction (typical attrition, churn, sales, production, maintenance, and internal costs)
- External prediction (typically external costs and framework conditions)
- New insights (through data exploration)
- Advice/ Recommendation
- Next best action
- Next best offer
- Next best product
- Next best interaction
- Real time data-driven interaction
- Text: Chatbot
- Audio: Personal Assistant like Siri or Alexa
- Next best action
Automation
Most often when you hear the concept of digitalization, you probably think about automation, i.e. reducing the need for manual work and intervention in the processes. In practice, this has been going on since the industrial revolution and is thus nothing new either strategically or technically.
What distinguishes AI-based automation from what has been going on for 200 years, however, is that it also includes operations that have previously required human assessments (interpretations). And this makes it possible to automate far more processes than before.
RPA is a form of rule motor that typically work across different systems and for many, there are big gains to be made in automating their processes using RPA, but it is not (necessarily) AI. But it can often provide more value to include AI in parts of the process, such as interpreting paper documents or images.
Interpretation of images, sound and text is very much a complicated operation and well within what we call AI. Often people have to come in to help the machine interpret better. This is called "supervised learning"
"Supervised" learning is often a key for learning complex categorizations. If, for example, the program is to distinguish between a picture of a dog or cat, it is essential that something or someone tells them whether it is guessing correctly, it must have a "right answer".
Image recognition is an extremely demanding exercise for an application, which is why this is often used as a test to distinguish between humans and robots in certain authentication solutions. It may not be so difficult to distinguish between a dog and a cat, but if the picture is of a random animal and the program is to guess which animal it is then it is an almost infinitely complex task. The well-known YouTube video "not hotdog" illustrates this problem well.
Automated categorization has been common to structured data for many years through e.g. data warehouse solutions, but categorizing unstructured data and large volumes in real-time presents other challenges that often require AI. For example, a somewhat more intelligent "tagging" of documents and emails through the use of so-called Natural Language Processing (NLP) could have major efficiency gains.
Physical robots are the most well-known AI-like technology in the past, and today they are far more ML-powered than ever before, both in the industry and eventually in the private market. For example, robot grass mowers are quite "stupid" as of today but will eventually be able to learn where the grass grows faster and mow more often there.
Insight
Automation is mostly about streamlining (and something about better quality), but it in itself offers little new insight. Traditionally, insights have a lot about exposing data from different parts of the business, putting these together, and giving you covariance and changes over time. This is what we would like to call Business Intelligence.
But traditional BI has its limitations when it comes to new insights. It requires you to know what you are looking for and it requires that data actually exists in a structured manner and is adapted.
With AI, some new opportunities are opening up to create completely new insights.
Forecasts
Forecasts are nothing new, it has been particularly central to financial management. But using AI, the forecasts can be far better for AI will be able to analyze parameters you previously didn't take into account (either because it was complicated or because you didn't know they mattered).
And it is possible to make forecasts in areas other than turnover and profit. Churn forecasts may be the best-known AI-based forecasts, but you can also forecast attrition, preventive maintenance and market development.
For many, more accurate forecasts are an important and long-awaited tool for better management of the business.
It is often just as important to have good forecasts of what is happening outside the business as it is internally. Today, far more external data is easily available and can be integrated with internal data and provide far more accurate forecasts.
Data exploration
Modern AI and data exploration (EDA) tools have accelerated what was previously called data mining.
Data exploration enables to find new interesting data as well as unknown patterns, categories and connections between data that can provide completely new insights about customers, internal conditions and the market in general.
Very little of the available data is currently available as input to analyses and reports, and with the extreme growth in data expected as a consequence of 5G and IoT, the gap between available data and data used for analysis purposes will widen sharply. There will therefore be an increasing need for tools that can distinguish between interesting and uninteresting data.
Advice / Recommendation
New insights and predictions do not by default provide some understandable basis for decision-making. And insight that do not potentially provide any new actions, is strictly speaking not very helpful.
What you usually need instead is suggestions for action based on the insight. And this is also an area AI can be of great help. And probably this area will eventually completely overshadow the other two, as this is where the real value lie.
Chatbot and PA
The most well-known AI-infected recommendation tool is probably the so-called Chatbots. But far from all chatbots being ML-based, most are probably still just rule engines. And therefore will not improve over time, but always give the same answer to the same question regardless of any feedback from the user.
On the other hand; a ML-based chatbot will accept the answers from users and use these in the assessment the next time the question is asked. Such "unsupervised" chatbots can get very good. But often supervision will help make these even more effective. Then some (most likely people) will explicitly say whether answers are good or bad and thus more quickly contribute to learning.
Chatbots are no longer just text-based and we find much of the same principles in Siri, Alexa, and other voice-controlled utilities (Personal Assistants), but these are often more general in usage and use a far more comprehensive register of info and links.
Next best action
A somewhat more advanced form of counseling is so-called NBA solutions. The best known here are probably “next best offer”- solutions used in personal online campaigns. The earliest well-known example here is Amazon's suggestion of books you should buy, nowadays it's probably rather personal adverts that pop up for ex. on facebook or on vg.no. And they will be becoming increasingly more accurate (and stop recommending products you have just purchased ...) and will eventually give you suggestions on products you didn't think you needed until you saw the commercial.
But in ordinary businesses, there are probably other NBA solutions that are just as important. Next best offer solutions are of course interesting in customer dialogues, but if we look ahead there are also other NBA solutions that are becoming common. For instance, to address the forecasts that were discussed under Insights and propose actions to address these. For instance:
What should we do to avoid churn and attrition?
- Which products should we invest more/less in the future?
- How to increase sales volume in specific geographic regions?
And AI will be able to help with this. It may not (at first) be able to propose completely new kinds of actions, but will be able to evaluate the effect of previously used actions and find what is best suited.
So. . where do I start?
- First of all; you don't start with AI, you start with a need or a challenge. It is possible that AI can solve this, but not have it as a prerequisite for taking the measures that are required.
- Secondly; do not try to run until you have learned how to crawl. The value of an advanced AI solution is often minimized if you do not have the more basic insight needs in place
- Shit in gives shit out. Especially with AI solutions where the processing of data is often cluttered, data quality and the right type of input data are essential.
- Choose the simple and low-hanging fruits first to get an idea of the potential and what it takes to realize this.
- Cost reduction or increased income? AI-solutions for cost reduction are often more low-hanging fruits with a clearer business case, but often the most valuable solutions are about increased income.
- By nature, an AI project can never be considered "finished". Since you are working to get P as close to 1 as possible, and P can never be 1, in principle you will never be completely finished. Instead, you have to define a cut-off, either on effort or on how good the model must be to be satisfied.
- Remember: AI requires maturation. One challenge with AI is that you don't always understand how output has emerged and you have to learn to trust this over time. Explainable AI (blog post) has a major focus at the moment and in the future, there will probably be demands for greater transparency in the AI models, but it will always be a question of trust and this will only be archived over time.